As part of our “Data Leaders” Interview Series, Leo Whyte (Head of Marketing at Genestack) caught up with Saulo Alves Aflitos (Senior Researcher Bioinformatics at Bejo) to discuss his thoughts on the future of data management.
Great to speak with you Saulo - Can you start by telling us a little bit about your role and how it fits within Bejo’s structure perhaps?
Sure, so I’m Saulo Aflitos and I'm a senior bioinformatician embedded in the Marker Technology and Genomics group at Bejo, a vegetable seed company located about 50 kilometers north of Amsterdam. The MT&Gs group is responsible for developing DNA-marker tests for our plants, specifically with the purpose to check seed on quality characteristics and to support our breeding processes by enabling making pre-selections on DNA-level. We have tons and tons of seeds coming through the company from the whole world, and we have to test each batch to give assurance that the seeds are genetically pure, have the right genetic identity and are true to type (no inbreds, no off types). Next to that a lot of effort is put in developing markers to follow traits.in the breeding program (MAS).
And that's particularly the hardest part when/where you try to identify markers for a trait and develop a test for it. First you need to find a variety which has the trait of interest (say resistance for disease for example). Then you need to find out where it is in the genome (genetic mapping). Then you can cross this material with your elite parent lines. When you cross the two, you want to keep all the great characteristics of your elite line plus the new trait. Nothing more, nothing less. In summary, you want to put in the minimum amount of DNA possible. This is most efficiently done by using DNA Markers and genomic information, therefore the name of the group. For each trait, and each elite line, we develop specific tests to introgress traits quickly by a process called MARBs. Those tests are then used to guide the breeding process to make it faster and more efficient. All of this while the breeders take care that the end product is still a great vegetable for the consumer. My job in this whole process is to give the MT&Gs researchers easy access to the data (reference genome, SNPs) that they need in order to develop their marker-assay.
So how do you envision your role and the way this is all done might change in the coming years?
Most of the time our traits are scored manually by a specialist and/or by breeders, but that, I hope, will be phased out in the future. New developments will make sure that we can start using more and more machines, cameras, machine learning or even regular pixel measurements, whenever necessary, practical and possible. This with the aim to be as precise and reproducible as possible and to have a higher throughput. Specialists and breeders will be responsible to train and verify those new methods besides grading immeasurable variables such as beauty and appeal, which are essential and highly subjective, as well as phenotypes which machines are just not able to measure.
At the Marker development front, I expect we will move from PCR tests to do whole genome sequencing more often. And I expect that all our main (parent) lines will have “reference grade” genome assemblies, not just comparisons to a golden reference. This is important because this means the volume of data will increase many folds and so will the quality of the data.
That’s an understandable but ambitious goal. You’ve been working with us for a few years now, how does data management and Genestack fit within that vision?
I need a place to start and that's where Genestack comes in. I have a lot of reference genome sequences and phenotypic data and we work with over 40 different crops. Each of them has between zero and 10 reference genomes sequenced, plus thousands of samples sequenced by RNA seq, genome sequencing, or were genotyped with arrays. etc. All that data has to be organised somewhere. We have a database and the searchable genomic files, but we had nothing centralised or searchable for the phenotypes.
So, two years ago I was introduced to Genestack at the Plant and Animal Genomics meeting in San Diego and we started talking. They showed a demo with the tomato genome and a hundred samples, and I was pleasantly surprised by the speed of the search for the phenotype and the capabilities to align the phenotype with all the other data measurements. Ultimately, it was exactly the system that I was about to make myself so if I don't have to make it myself then that's less maintenance for us as well as having back-up support and ongoing development we don’t have to worry about. We are a group of just four developers who do the programming. We have to do a lot of analysis also to help our colleagues. So, development time is limited, and this allows us to focus on the real meat of our roles rather than developing and maintaining a new system. So now we have Genestack software installed. It was a great experience, we gave Genestack access to some virtual machines in our private cloud and a few days later it was installed, completely containerised. Now we are loading the first data into the system and are giving it to the researchers to see if we can make their life easier. The Genestack services team are also helping us with that part. I give them the data and they load it for us, saving us even more time.
So like most companies I’m sure Bejo is working with public data and in consortia, do you think this type of centralised data management system could be useful in those situations as well?
Yes, we do use public data and are members of many consortia with several companies and universities. “Potentially” is the simple answer. Previously with most of the consortia there was nothing in terms of data management, everything was just in flat files. Consortia have become wise to the need for FAIR data management, and recently it became Dutch Law. Understanding that at every level data has to be made FAIR, not only to publish, but also has to be searchable. People don’t want to be scrambling trying to figure out what the data means and how to use it.
There have been a few attempts at a database, but it is more of a file identification catalogue than a proper database. So yeah, maybe it would be interesting to have a Genestack instance for these things but there are a few limitations you have to think of too.
Firstly, you would need a lot of flexibility around longitudinal data and matching measurements for multiple different experiments over time from different sources. Often when these academic experiments are being done there isn’t the same level of strictness on the longer-term plan, so the system needs to be flexible enough to cope with the slight chaos that comes with that.
The second constraint to think of is that when you are part of a consortium, universities involved will ultimately publish so they have to stick to a standard and use standard ontologies. We get the data, like it or not, using those standards and not to whatever standard we use in our internal databases. If we don't want to spend a lot of time always having to convert back to our proprietary ontologies, then the best route for us is to standardise using the same standards as the academy and extend it to our own needs. This also helps to avoid a lot of common problems around nomenclature between different branches of the field, let's say, farmers in the field versus the nomenclature for agronomists and biologists. Each one of our crops might give a different name to the same phenotype, just because that's the correct way to call that type of leaf in lettuce versus cabbage.
Without a standardised central repository there is a risk that the system would miss something as it would be named using different terms when, biologically, they are the same and therefore genetically, they might've come from the same components. Therefore, for me, I want to be sure that it is stored with the biologically correct name in my database so that we can maybe identify the underlying genetic component responsible for a given phenotype in each crop. It does not matter what you call a phenotype when you are selling the vegetable in the supermarket or what you call it when talking with the farmers, those names are only aliases to the botanical name. This distinction can help with identifying new traits by always being able to correlate the phenotypes with genotypes, independent of how the phenotype is called in different backgrounds. That's the main reason why I personally want to have a database that links phenotype and genomic data.
So yeah, I think the idea of better data management in consortia is important and maybe Genestack could support it, something worth exploring for sure.
So, I suppose that leads on to our final question - if you met someone and they were just at the start of trying to work out how to manage their data and decide what data management looks like for them, what would your advice be?
My advice is to examine what you plan to do with the data and choose a kind of structure that allows that, with built in room for scalability in the model.
You may have a need for two separate, but inherently similar, systems. One for the more chaotic one-off experiments where each experiment can be found and used immediately upon creation and where data will likely not be used again in the future. You can load the data there to keep it safe, but that’s one time use data. You want complex data, such as genomic data, to be well-structured and FAIR but your experimental data is likely to lack standardisation.
Then you need a stricter reference database where all your reference materials are well characterised. That’s where your markers should be stored, where your de novo assemblies and the deep sequences of parental lines should be stored. A repository, where you want people to be able to find which materials they can start a project with by searching for their trait of choice (for example resistance) and for divergent genetics because often you don’t want to do experiments with materials which are related to each other. They can use this database to search and get reliable, high quality reference samples. This should be strictly controlled and organised with standardised ontologies and nomenclature. That's my recommended design anyway.
From there, work out what calculations you need to do. Genestack is not a system that does calculations per se, it’s about finding the data that can be fed into appropriate apps or tools downstream via API or whatever mechanism you’re looking for. It’s designed for combining phenotypic and measurement data to make it FAIR. When you’re thinking about what their goal is, also make sure you’ve implemented the right tool for the job you’re trying to do!
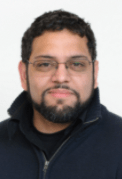
Saulo Aflitos has a PhD in Bioinformatics from Wageningen University, The Netherlands, and has worked at Bejo since 2017.
Bejo is a leading company in breeding, production and sales of vegetable seeds. With operations in more than 30 countries, we are an internationally oriented family business. Our 1,900 employees are dedicated to developing the best vegetable varieties for the present and the future.
Genestack accelerates the speed to breakthrough in Life Sciences by unlocking the power of data. Our Data curation, management and search platform help your teams be more effective, efficient and impactful in their research by reducing redundancy in experiments and increasing the usability of your existing legacy and public data. Learn more at www.genestack.com or contact sales@genestack.com